The Future of Collections for Wireless Carriers/Telcos
Best practices and recommendations for more efficient, personalized collections strategies
Michael Fife VP Sales & Consulting, US, Provenir
Sam Rohde Director, PreSales, North America, Provenir
Andy Beddoes Principal Consultant, North America, Provenir
Collections activities enormously impact the financial performance of U.S.-based wireless carriers. There are 1%-5% of all U.S. subscriber accounts in delinquency at any given time. And with over 450 million post-paid wireless accounts active in the U.S. and an average past due balance between $200 and $300, that means there are over $3 billion dollars that are past due and at risk. To combat these startling stats, wireless carriers need to take advantage of new innovations in advanced analytics and holistic, cloud-native risk decisioning solutions to execute best-practice treatments before consumers go past due. Telcos that deploy advanced analytics to get ahead of payment risks see up to a 10% improvement in recovery rates when compared to those who use legacy processes and static scorecard methods.
Adopting these newer innovations and best practices can drastically reduce operating costs within your collections functions and also increase returns on collections activities. The ease with which internal and third-party data sources can be integrated and orchestrated, and the ease with which advanced analytics can be set up, tested and promoted to production, are primary drivers of these returns on investment.
So we’re looking at exactly what these best practices are for pre-collections and collections decisioning, and what has worked for large telco organizations around the globe.
Looking at best practices from telco companies around the world reveals that a collections risk decisioning strategy for wireless carriers should consist of at least seven key components. And the platform upon which these are configured and executed must allow simple, self-service access for business users to set up, test, and deploy each component without added burden on tech teams or IT.
- Champion / Challenger: Can you implement independent and in-stream testing of objects that execute further down in a flow? An unlimited random number generator that divides decisioning down two or more flows allows for complex testing strategies to be executed, which is important for fine tuning the impact of strategies on collected balances and is a best-practice first step.
- Calculation of Attributes: Be sure you can enable the ingestion of internal and external data to calculate attributes such as days past due, debt-to-income, skip trace required, and other variables useful in predicting behavior and best treatments.
- Reasons for Collections: The third critical component is being able to calculate internal data that is useful for segmentation, including but not limited to billing cycle data, promise-to-pay broken, skip trace required, and other attributes.
- Portfolio Segmentation: Can you execute portfolio segmentation in real-time, based on the data your decision engine has ingested to determine the appropriate collections stage (early, mid, late, or more divisions) and subsequent actions?
- Configurable Collections Stages: Ensure the creation of configurable, divided collections stages where distinct actions and treatments can be executed based on the segmentation characteristics that were executed in the previous step.
- Scoring Models: The ability to test and deploy advanced analytics that drive the treatments are crucial to successfully increasing balances collected. These include everything from behavioral scorecards and roll-rate models, to risk grades and proposed settlement amounts, that inform the best communication channels, timing, tone, offers and other actions.
- Treatments: Each of these previous steps lead to you being able to automatically push actions through existing communication channels (SMS, email, push notification, phone, etc.), informing the tone, the settlement offer, and other iterative actions that drive collected balances. Because not all channels elicit the best response – for example, 73% of Gen Z consumers say SMS is best for reminding when payments are past due. This is where the use of advanced analytics can help, informing the right options for individual customers.
Modern, cloud-native risk decisioning solutions allow business users to administer the creation and testing of individual decisioning objects or nodes. These nodes interact with each other either concurrently or sequentially and range in complexity from simple business rules to advanced analytics, which users can then create and manage through a low-code interface to improve returns on collections activities. Additionally, decisioning software that is user friendly reduce the technical burden and operating costs of the collections function. What does this mean? In short: business users must be able to manage the end-to-end flow in both test and production environments without having to involve IT.
Here’s an example of a best-practice collections decisioning workflow, which comes from dozens of large-scale implementations thanks to the subject matter expertise of risk and collections professionals. They created this end-to-end sequence for wireless carriers to use, and it can be modified as necessary to adapt to different requirements in order to efficiently execute next-best treatments.
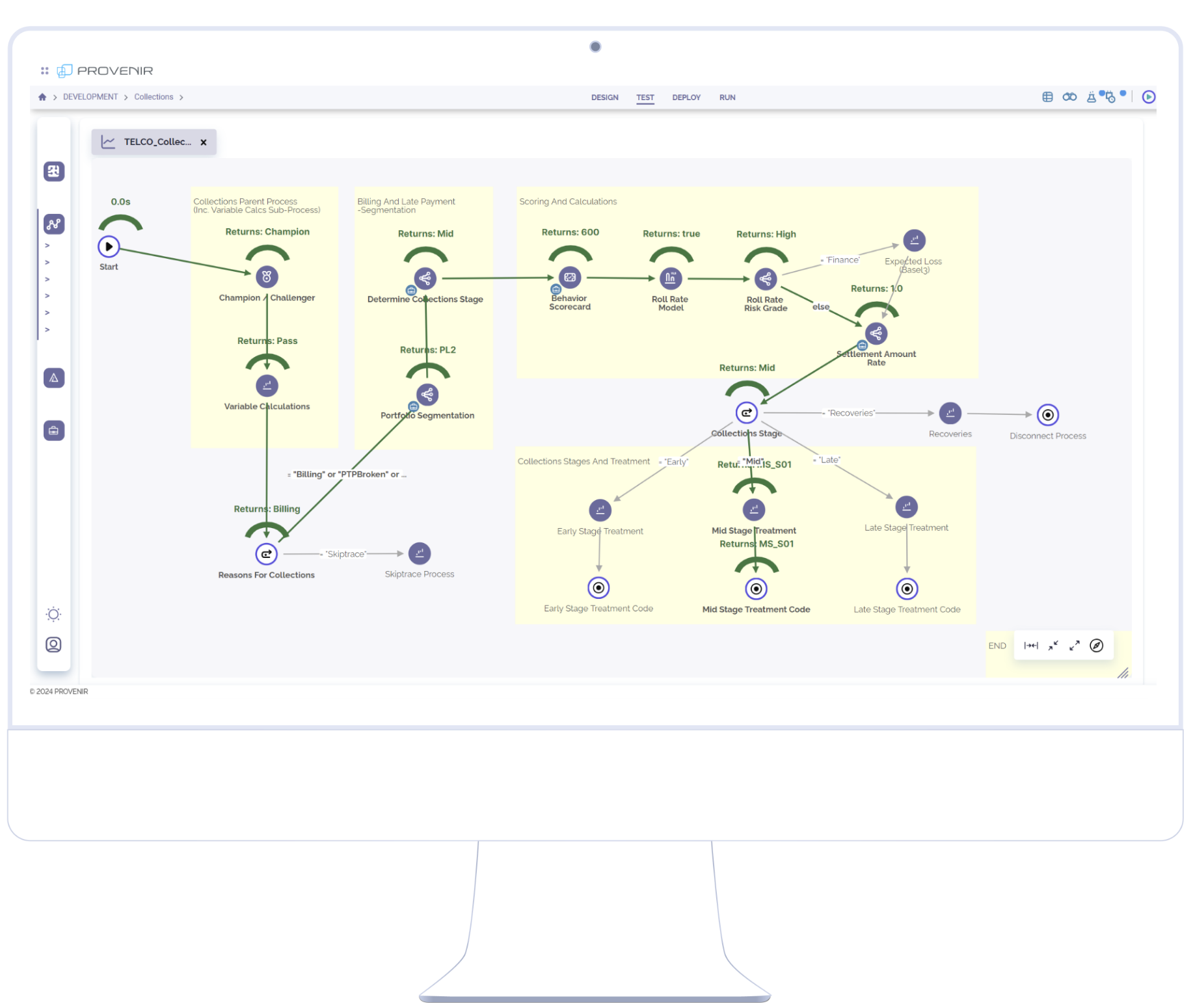
The workflow pictured above uses a combination of on-us behavior data, off-us behavior data from 3rd parties such as credit bureau and speciality telco data, previous contact history data, and socio-demographic data. All of these combine to build a holistic, comprehensive view of a delinquent customer, as outlined in the seven components we described.
- On-us behavioral data includes the customer’s payment history, delinquency history, and returned checks, among other attributes.
- Off-us behavioral data involves third-party data sources that provide insights into a customer’s financial obligations and commitments, as well as updates on their behavior based on almost real-time updates.
- Previous contact history data is critical in learning from previous collection contact attempts and modifying the treatment approach accordingly.
- Socio-demographic data can be used to build customer profiles to assist in selecting the appropriate channel of communication.
Leveraging these various data sources and applying advanced analytics such as random forest or XGBoost machine learning techniques to predict behavior, propose settlement amounts, and to gauge time and channel preferences allows collection teams to build a more targeted, personalized approach to collections, based on customer preferences and circumstances.
Making a significant departure from more traditional, legacy processes (which often rely on core static classifications such as days past due or single risk scores), this new approach highlights a more modern, individualized way of ensuring efficient, effective collections strategies. By evolving beyond logistic regression and decision trees to next-generation collections models that lean on machine learning (which learns from previous nodes within its model construct), the final customer treatment is much more personalized, focused on outcomes and response propensity.